Note
Go to the end to download the full example code
Denoise images using Local PCA via empirical thresholds#
PCA-based denoising algorithms are effective denoising methods because they explore the redundancy of the multi-dimensional information of diffusion-weighted datasets. In this example, we will show how to perform the PCA-based denoising using the algorithm proposed by Manjón et al.[1].
This algorithm involves the following steps:
First, we estimate the local noise variance at each voxel.
Then, we apply PCA in local patches around each voxel over the gradient directions.
Finally, we threshold the eigenvalues based on the local estimate of sigma and then do a PCA reconstruction
To perform PCA denoising without a prior noise standard deviation estimate please see the following example instead: Denoise images using the Marcenko-Pastur PCA algorithm
Let’s load the necessary modules
from time import time
import matplotlib.pyplot as plt
import numpy as np
from dipy.core.gradients import gradient_table
from dipy.data import get_fnames
from dipy.denoise.localpca import localpca
from dipy.denoise.pca_noise_estimate import pca_noise_estimate
from dipy.io.gradients import read_bvals_bvecs
from dipy.io.image import load_nifti, save_nifti
Load one of the datasets. These data were acquired with 63 gradients and 1 non-diffusion (b=0) image.
dwi_fname, dwi_bval_fname, dwi_bvec_fname = get_fnames(name="isbi2013_2shell")
data, affine = load_nifti(dwi_fname)
bvals, bvecs = read_bvals_bvecs(dwi_bval_fname, dwi_bvec_fname)
gtab = gradient_table(bvals, bvecs=bvecs)
print("Input Volume", data.shape)
Input Volume (50, 50, 50, 64)
Estimate the noise standard deviation#
We use the pca_noise_estimate
method to estimate the value of sigma to be
used in the local PCA algorithm proposed by Manjón et al.[1].
It takes both data and the gradient table object as input and returns an
estimate of local noise standard deviation as a 3D array. We return a
smoothed version, where a Gaussian filter with radius 3 voxels has been
applied to the estimate of the noise before returning it.
We correct for the bias due to Rician noise, based on an equation developed by Koay and Basser[2].
Sigma estimation time 1.9994478225708008
Perform the localPCA using the function localpca#
The localpca algorithm takes into account the multi-dimensional information
of the diffusion MR data. It performs PCA on a local 4D patch and
then removes the noise components by thresholding the lowest eigenvalues.
The eigenvalue threshold will be computed from the local variance estimate
performed by the pca_noise_estimate
function, if this is inputted in the
main localpca
function. The relationship between the noise variance
estimate and the eigenvalue threshold can be adjusted using the input
parameter tau_factor
. According to Manjón et al.[1], this
parameter is set to 2.3.
Time taken for local PCA (slow) 87.76140713691711
The localpca
function returns the denoised data which is plotted below
(middle panel) together with the original version of the data (left panel)
and the algorithm residual image (right panel) .
sli = data.shape[2] // 2
gra = data.shape[3] // 2
orig = data[:, :, sli, gra]
den = denoised_arr[:, :, sli, gra]
rms_diff = np.sqrt((orig - den) ** 2)
fig, ax = plt.subplots(1, 3)
ax[0].imshow(orig, cmap="gray", origin="lower", interpolation="none")
ax[0].set_title("Original")
ax[0].set_axis_off()
ax[1].imshow(den, cmap="gray", origin="lower", interpolation="none")
ax[1].set_title("Denoised Output")
ax[1].set_axis_off()
ax[2].imshow(rms_diff, cmap="gray", origin="lower", interpolation="none")
ax[2].set_title("Residual")
ax[2].set_axis_off()
plt.savefig("denoised_localpca.png", bbox_inches="tight")
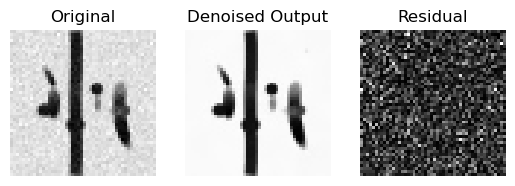
Below we show how the denoised data can be saved.
The denoised data is saved in the same format as the input data.
save_nifti("denoised_localpca.nii.gz", denoised_arr, affine)
References#
Total running time of the script: (1 minutes 32.087 seconds)