Note
Go to the end to download the full example code
MultiTensor Simulation#
In this example we show how someone can simulate the signal and the ODF of a single voxel using a MultiTensor.
import matplotlib.pyplot as plt
import numpy as np
from dipy.core.gradients import gradient_table
from dipy.core.sphere import HemiSphere, disperse_charges
from dipy.data import get_sphere
from dipy.sims.voxel import multi_tensor, multi_tensor_odf
from dipy.viz import actor, window
For the simulation we will need a GradientTable with the b-values and
b-vectors. To create one, we can first create some random points on a
HemiSphere
using spherical polar coordinates.
rng = np.random.default_rng()
n_pts = 64
theta = np.pi * rng.random(size=n_pts)
phi = 2 * np.pi * rng.random(size=n_pts)
hsph_initial = HemiSphere(theta=theta, phi=phi)
Next, we call disperse_charges
which will iteratively move the points so
that the electrostatic potential energy is minimized.
hsph_updated, potential = disperse_charges(hsph_initial, 5000)
We need two stacks of vertices
, one for every shell, and we need two sets
of b-values, one at 1000 \(s/mm^2\), and one at 2500 \(s/mm^2\), as we discussed
previously.
vertices = hsph_updated.vertices
values = np.ones(vertices.shape[0])
bvecs = np.vstack((vertices, vertices))
bvals = np.hstack((1000 * values, 2500 * values))
We can also add some b0s. Let’s add one at the beginning and one at the end.
bvecs = np.insert(bvecs, (0, bvecs.shape[0]), np.array([0, 0, 0]), axis=0)
bvals = np.insert(bvals, (0, bvals.shape[0]), 0)
Let’s now create the GradientTable
.
gtab = gradient_table(bvals, bvecs=bvecs)
In mevals
we save the eigenvalues of each tensor.
mevals = np.array([[0.0015, 0.0003, 0.0003], [0.0015, 0.0003, 0.0003]])
In angles
we save in polar coordinates (\(\theta, \phi\)) the
principal axis of each tensor.
angles = [(0, 0), (60, 0)]
In fractions
we save the percentage of the contribution of each tensor.
fractions = [50, 50]
The function multi_tensor
will return the simulated signal and an array
with the principal axes of the tensors in cartesian coordinates.
We can also add Rician noise with a specific SNR.
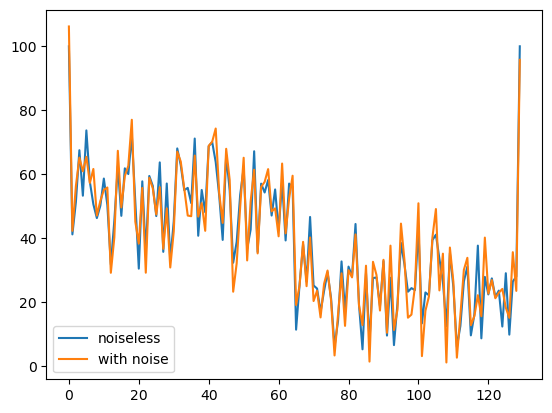
Simulated MultiTensor signal
For the ODF simulation we will need a sphere. Because we are interested in a simulation of only a single voxel, we can use a sphere with very high resolution. We generate that by subdividing the triangles of one of DIPY’s cached spheres, which we can read in the following way.
sphere = get_sphere(name="repulsion724")
sphere = sphere.subdivide(n=2)
odf = multi_tensor_odf(sphere.vertices, mevals, angles, fractions)
# Enables/disables interactive visualization
interactive = False
scene = window.Scene()
odf_actor = actor.odf_slicer(odf[None, None, None, :], sphere=sphere, colormap="plasma")
odf_actor.RotateX(90)
scene.add(odf_actor)
print("Saving illustration as multi_tensor_simulation")
window.record(scene=scene, out_path="multi_tensor_simulation.png", size=(300, 300))
if interactive:
window.show(scene)
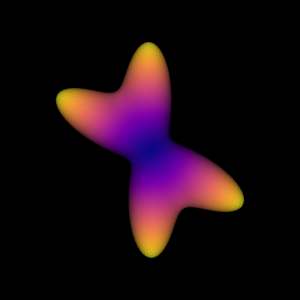
Saving illustration as multi_tensor_simulation
Simulating a MultiTensor ODF.
Total running time of the script: (0 minutes 3.650 seconds)