Note
Go to the end to download the full example code
Reconstruct with Diffusion Spectrum Imaging (DSI)#
We show how to apply Diffusion Spectrum Imaging [1] to diffusion MRI datasets of Cartesian keyhole diffusion gradients.
First import the necessary modules:
import matplotlib.pyplot as plt
import numpy as np
from dipy.core.gradients import gradient_table
from dipy.core.ndindex import ndindex
from dipy.data import get_fnames, get_sphere
from dipy.io.gradients import read_bvals_bvecs
from dipy.io.image import load_nifti
from dipy.reconst.dsi import DiffusionSpectrumModel
from dipy.reconst.odf import gfa
Download and get the data filenames for this tutorial.
img contains a nibabel Nifti1Image object (data) and gtab contains a GradientTable object (gradient information e.g. b-values). For example to read the b-values it is possible to write print(gtab.bvals).
Load the raw diffusion data and the affine.
data, affine, voxel_size = load_nifti(fraw, return_voxsize=True)
bvals, bvecs = read_bvals_bvecs(fbval, fbvec)
bvecs[1:] = bvecs[1:] / np.sqrt(np.sum(bvecs[1:] * bvecs[1:], axis=1))[:, None]
gtab = gradient_table(bvals, bvecs=bvecs)
print(f"data.shape {data.shape}")
data.shape (96, 96, 60, 203)
This dataset has anisotropic voxel sizes, therefore reslicing is necessary.
Instantiate the Model and apply it to the data.
Let’s just use one slice only from the data.
dataslice = data[:, :, data.shape[2] // 2]
dsfit = dsmodel.fit(dataslice)
Load an odf reconstruction sphere
sphere = get_sphere(name="repulsion724")
Calculate the ODFs with this specific sphere
C:\Users\skoudoro\AppData\Local\Continuum\Anaconda3\envs\py310\lib\site-packages\dipy\reconst\dsi.py:172: RuntimeWarning: invalid value encountered in divide
Pr /= Pr.sum()
ODF.shape (96, 96, 724)
In a similar fashion it is possible to calculate the PDFs of all voxels in one call with the following way
PDF.shape (96, 96, 17, 17, 17)
We see that even for a single slice this PDF array is close to 345 MBytes so we really have to be careful with memory usage when use this function with a full dataset.
The simple solution is to generate/analyze the ODFs/PDFs by iterating through each voxel and not store them in memory if that is not necessary.
for index in ndindex(dataslice.shape[:2]):
pdf = dsmodel.fit(dataslice[index]).pdf()
If you really want to save the PDFs of a full dataset on the disc we
recommend using memory maps (numpy.memmap
) but still have in mind that
even if you do that for example for a dataset of volume size
(96, 96, 60)
you will need about 2.5 GBytes which can take less space
when reasonable spheres (with < 1000 vertices) are used.
Let’s now calculate a map of Generalized Fractional Anisotropy (GFA) [2] using the DSI ODFs.
GFA = gfa(ODF)
fig_hist, ax = plt.subplots(1)
ax.set_axis_off()
plt.imshow(GFA.T)
plt.savefig("dsi_gfa.png", bbox_inches="tight")
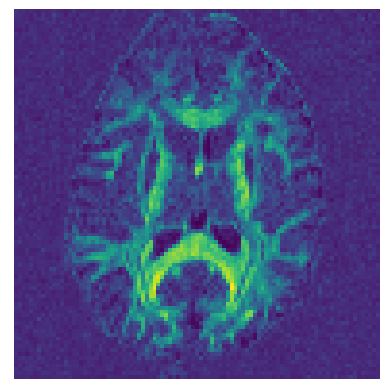
See also Calculate DSI-based scalar maps for calculating different types of DSI maps.
References#
Total running time of the script: (0 minutes 30.412 seconds)